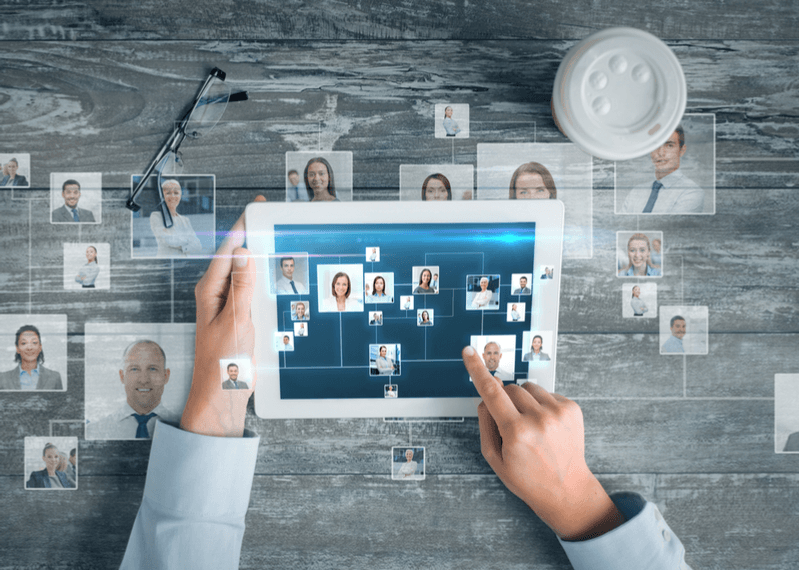
One approach to network threats is to monitor active data streams – in the event of an anomaly, an alarm is sounded. However, this approach has proven unsuccessful in practice because such solutions ignore the biggest risk factor in the network: the user.
A user-centric approach to network security solutions is ahead of normal surveillance solutions. So he can provide answers to such critical questions as: Who accesses the network? What does the user access? Is this access happening within the normal behavior of this user?
Who exactly accesses the network?
The idea of tracking who accesses the network may sound banal. In fact, this is becoming increasingly difficult in practice today because most employees have complex identities that consist of a multitude of user accounts, applications, and folders under their names. Even in a midsize business, an employee’s identity can include a standard Windows ID and numerous other accounts for applications such as SAP, Salesforce, and Oracle, just to name a few. Adding to this confusion is also the rise in BYOD policies, which means many employees are using personal devices in the corporate network. Therefore, it is extremely difficult to effectively manage each ID for each employee in a central location. It gets even harder
So how can a company assign the use of all services to a particular user when there is no way to assign them correctly? Without a way to answer these questions, it’s impossible to tell who’s accessing the network.
What exactly is being accessed?
Just as monitoring users seems easy at first glance, tracking what is being accessed can be simple and should be a pretty simple part of everyday network security. In fact, this is rarely the case. In many companies, one does not know exactly what is stored and accessible on the network. This is usually due to the lack of a central asset monitoring system. If you do not know what kind of data you have, it’s difficult to keep track of whether it’s being accessed.
The reason for such a trivial mistake is usually that IT security systems were built piecemeal over time. This has resulted in a variety of different solutions that at first glance perform a similar task, but all with limited functionality. This means that the IT department can know which server is being accessed and which employee is accessing it. However, it is unlikely that the IT team knows what other information is on the same server or how sensitive it is.
Is the user behavior normal?
Even if the IT team is able to effectively track who is accessing the network connections and what exactly, the question of whether it is “normal behavior” for the person concerned can be extremely difficult to answer. This is because the context required to effectively assess user behavior is not only captured by the network flow data. As such, it is often little more than a well-founded guess as to whether a person is within the bounds of what is considered “normal” or whether their actions are abnormal and therefore suspicious.
Machine learning gives wings to IT security
Terms such as “data science” or “machine learning” began long ago as empty buzzwords in the IT industry. For some time, however, the clever algorithms have been helping to identify patterns in many areas – and have great potential for doing so in IT security. So, security experts want machine learning to help answer the data access questions above. This desire has come true, and machine learning, when properly used, can discover important connections between seemingly disconnected pieces of identities. This gives IT security teams a detailed overview of a user’s activities, even though different identity components are not explicitly linked.
The missing link: the context
A classic example: An employee, for example, logs into the network at the office using his personal access data. Later, he logs on to a personal device from home with an admin account. Normally these two actions would not be linked to the same identity. Using behavioral data, solutions based on machine learning can not only link them together, but also track employee actions over time, providing a comprehensive view of their actual network activity.
With the help of machine learning algorithms, trends can be analyzed in this way and normal behavior profiles per user can be created. This helps to create the much-needed context to recognize and label any activity that deviates too far from what is considered acceptable or normal. In addition, various machine learning techniques can be used to create accurate network asset models that give IT teams an accurate picture of everything on the network. This makes it much easier to keep track of what is being retrieved at a given time. Accordingly, data from executives and board members may be labeled as “high risk,” meaning they will be subject to stronger scrutiny and/or stricter security measures.
Effective network security is not optional, is absolutely critical.
In principle, the threat of cyber attacks increases daily and effective network security is very important for businesses. Using the right solutions helps every business understand exactly who is accessing the network, what they are doing, and whether they should do it. Machine learning will play an important role by not only linking key information in a way that was previously not limited to monitoring network traffic, but also providing IT teams with the context they need make informed security decisions.
Contact us today to learn about Bleuwire™ services and solutions in how we can help your business.